Latest Process Mining Functionality, Challenges, and Future Evolutionary Trends
English follows Japanese.
今回の記事では、2021年夏時点における、プロセスマイニングのテクノロジーやソリューションに焦点を当て、機能、課題、今後の進化についてお伝えします。
1 プロセスマイニングの最新機能群
プロセスマイニングは、テクノロジーやツールの側面に関心が行きがちであるが、その本質は、データ分析の理論体系・方法論(Discipline)である。実際、プロセス“マイニング”という言葉でわかるように、データマイニングの一類型と考えることができる。ただし、あらゆる事象を分析対象とする幅広い概念のデータマイニングと異なり、文字通り「プロセス」を分析対象とするのがプロセスマイニングである。その基本となる用途は「プロセスの可視化」であり、プロセスが可視化されたことによって、対象プロセスがはらむ問題点の発見が容易になる。結果として、プロセス改善の取り組みに大きな役割を果たすことができる。
1.1 現在の主要機能
さて、プロセスマイニングは、前述したように「プロセスの可視化」の方法論の確立とツール開発からその研究がスタートしている。それは、業務遂行に使用するITシステムから抽出されたデータに基づき、業務手順を示すフローチャートを自動的に作成する機能であり、「プロセス発見(Process Discovery)」と呼ばれる。その後、研究の進展、ツールの高度化に伴い、様々な機能が実装されてきた。以下は、現在のプロセスマイニングツールの多くが実装している主な分析機能である。
・プロセス発見:
業務手順を自動的にフローチャート化し、作業頻度や所要時間などを算出する
・適合性検査:
データに基づき発見された現状プロセス(as-is)と標準プロセス(to-be)との比較分析を行い、現状プロセスの逸脱を抽出する
・ダッシュボード:
対象プロセスについて、様々な切り口から集計・分析した結果を各種グラフや図でビジュアルに表示する(BIツールと同等)
1.2 最新機能群
さらに、近年では、最先端のプロセスマイニングツールでは、次のような最新機能群が搭載され始めている。
・ビジネスルールマイニング:
対象プロセスにおいて、フローの分岐(意思決定ノード)が発生している箇所がある場合、その分岐を決定している基準=ビジネスルールをデータに基づいて自動発見する
・シミュレーション(What-If分析)
プロセス発見機能によって可視化された現状プロセスについて、一部のタスクを排除したり、あるいは自動化したりすることで、どの程度の改善効果が期待できるかをシミュレートする
・運用サポート
現在仕掛中の案件について、業務遂行に関わるデータをリアルタイムに吸い上げ、業務の逸脱を探知したり、将来の問題発生を予測したりして、担当者にアラートを出す、また最善手を提案する、あるいは自動的に改善施策を実行する。
上記3つの最新機能のうち、ビジネスルールマイニング、およびシミュレーションは、既に完了した案件、すなわち過去データを分析対象としているが、運用サポートは、未完了の案件に関わるデータを逐次処理し、円滑な業務遂行を支援することが主眼である。この意味で、運用サポートは、分析の方法論の枠を超えたITソリューションの一形態とも言えるだろう。このため、プロセスマイニング業界最大手のセロニス社では、当該機能を「EMS(Execution Management System)」と呼んでいる。
2 プロセスマイニングが克服すべき課題
2.1 データ前処理の難しさ
データマイニングでは、全体の所要時間の約8割がデータの収集・抽出、クリーニングといったデータ前処理に費やされると言われる。プロセスマイニングでも同様である。多様なITシステムから抽出された数十~数百に及ぶデータファイルを適切に統合し、抜け漏れ、文字化けなどのダーティなデータを補正し、ツールに投入して分析可能な「データセット」を作り上げる労力は大きい。プロセスマイニングにおけるデータ前処理の難度を高くしている要因としては、データの抽出元が各種業務システムであることから、業務システムへの理解が必要であること、また、業務プロセス改善に資する分析結果を導くためのデータセットを作成するためには、業務自体への理解、また業務改善手法にもある程度通暁している必要があることが挙げられる。
2.2 ツールの分析品質
分析品質については2つの課題を述べたい。一つはDFGs(Directly Follows Graphs)の限界、もうひとつは、Convergence/Divergence問題である。
2.2.1 DFGsの限界
プロセスマイニングの基本機能である「プロセス発見」は、当初、ペトリネットがベースになっていたが、より現実に近いフローチャートを再現するために、様々なアルゴリズムが開発されてきている。ただ、業界有識者の話によれば、現在実用化されているプロセスマイニングツールのほとんどは、ファジーマイナーと呼ばれるアルゴリズムに基づいたもの(各社独自の改善は行っていると思われる)であると言われている。
同アルゴリズムは、一般にDFGs(Directly-follows Graphs)と呼ばれる。ペトリネットや、また業務手順をフローチャートとして記述するための世界標準であるBPMN(Business Process Modeling and Notation)と異なり、ノードとノードが直接(Directly)結びつけられたフローチャートがDFGsである。すなわち、分岐ノードが描かれないため、このアルゴリズムでは、どこでどのような分岐が発生しているのか、具体的には、排他的(OR)なのか、並行的(AND)なのか、といったことが把握できない。このため、現状のプロセスを自動的に再現するとはいっても、分岐が明確でない不完全なものになるというのが現実である。もちろん、これについては、BPMN形式のフローチャートへの自動変換や、前述したビジネスルールマイニングの採用などの機能改善が行われてきている。

図1 Petri net、BPMN、Fuzzy Minerのフロー図例
上図でわかるように、DFGsであるFuzzy Minerには、Petri netやBPMNのような分岐ノードが存在しないため、同じプロセスの表現でありながら、Fuzzy Minerでは分岐のルールを判別することができない。
2.2.2 Convergence/Divergence問題
プロセスマイニングでは、対象プロセスで処理される案件に対して行われる各アクティビティを束ねて、フローチャートを描くために、「案件ID」、「アクティビティ(処理内容)」、およびタイムスタンプの3項目が必須である。例えば、請求書処理プロセスであれば、各請求書に付番されている個別の請求書番号、そして、その請求書に対して行われる「受領」、「確認」、「承認」、「支払い」などのアクティビティをタイムスタンプとともにITシステムから抽出することになる。
実際のプロセスにおいてしばしば直面するのは、案件IDがひとつではないという点である。具体例を示そう。図2は、エンジニアリング会社の受注から資材調達までのプロセスの一般的なイメージである。受注した機械は、発注企業の仕様に基づいて製造されなければならないため、受注後は、まず設計を行い、次に設計図(Blueprint)に基づいて必要な資材・パーツを洗い出し、サプライヤに発注する流れとなる。ここで、受注した案件は、工事番号(Construction Number)で管理されるが、一つの機械に対して複数の設計図が作成されるため、設計段階では、設計図番号(Blueprint Number)が用いられる。さらに、資材・パーツの洗い出しにはパーツ番号(Parts Number)が、調達時には、複数のパーツがいくつかにまとめられて調達要求が出される。この時は、調達要求番号(Procurement Request Number)が付番される。さらに、複数の調達要求は、サプライヤ毎に集約されて発注が行われる。ここでは発注番号(Order Number)が管理用のIDとなる。
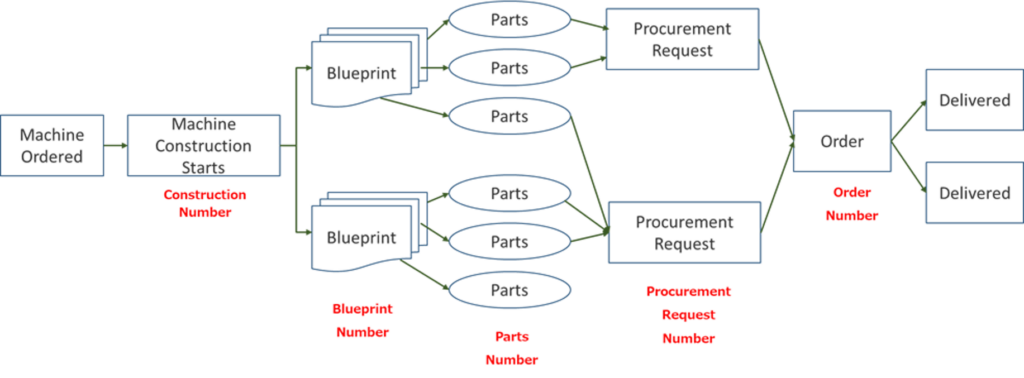
図2 受注から資材調達までのプロセス例(エンジニアリング会社)
1台の機械受注に対して複数のBluleprint、Parts、Procurement Request、Orderが紐づけられ、ひとつの案件IDだけでは適切な分析が行えない
このように、ひとつの案件が処理されていく中で、集約されたり(Convergence)、拡散したり(Divergence)するプロセスが実務ではごく普通に見られる。従来のアプローチでは、プロセス開始時の工事番号を案件IDとして資材調達までを一気通貫に分析することになるが、途中に集約や拡散が存在していると、実態とはかけ離れたプロセスが再現されてしまう。(例えば、拡散している箇所は単なる繰り返しタスクとして認識されるなど)
このConvergence/Divergence問題は、プロセスマイニングの分析品質を左右する最大の課題と言える。そこで、近年では、プロセスマイニングのゴッドファーザー、Wil van der Aalst教授が率いる研究者たちが「Object-Centric Process Mining」(1)と称する独自の方法論により当課題の解決に取り組んでいる。 また、myInvenioには、マルチレベルマイニングという機能が実装されており、一つのプロセスについて複数の案件IDを設定することで、プロセスの集約・拡散の状況を加味したフローの再現を実現している。
3 今後の進化の方向性
プロセスマイニングは、データ分析の枠を超えて、業務支援ソリューションとしての役割も果たしつつあることは前述した。ここでは、プロセスマイニングは今後、どのように進化していくのか、俯瞰的な視点で述べてみたい。
3.1 プロセスマイニング1.0
プロセスマイニングは。現状のプロセスをデータから自動再現する「プロセス発見」が基本機能であった。これは、現状をありのままに描きだすという点において「記述的分析(Descriptive Analysis)」である。
ただし、本来やりたいことは、プロセスに潜む非効率性やボトルネックなどの問題個所の抽出である。つまり、どこが悪いのか、を探し出さなければならない。そこで、この部分の処理時間が長すぎる、あるいは繰り返しが多いなど、容易に問題と思われる個所を教えてくれる機能が付加されている。診断的分析(Diagnostic Analysis)に属する機能である。プロセスマイニングツールでは、一般に「根本原因分析(Root Cause Analysis)」と命名されている。
以上は、過去データを対象とする分析機能であり、プロセスマイニング1.0と呼ぶべきものであろう。

図3 プロセスマイニングの進化
プロセスマイニングの機能は、プロセスマイニング1.0から2.0へと大きく進化しつつある
3.2 プロセスマイニング2.0
プロセスマイニングの分析対象として、未完了、すなわち現在進行中の案件データをリアルタイムに取り込むようになると、逸脱の発見に加えて、現在走っている案件はあとどのくらいで完了しそうなのか、といった所要時間の予測や、将来に発生するかもしれない逸脱の予測も可能になる。こうした予測的分析(Predictive Analysis)が実装されたツールも増えつつある。
さらには、予測結果に基づいて、所要時間を短縮するために、あるいは将来の逸脱発生を未然に防ぐために、今どのような対応を行うべきかを提案する機能を持つツールも登場しつつある。これは「処方的分析(Prescriptive Analysis)」の機能である。
こうした未完了データを扱うプロセスマイニング分析は、既存のプロセスマイニング1.0を大きくバージョンアップするものであり、プロセスマイニング2.0と呼ぶことができるであろう。
予測的分析、処方的分析は未成熟であり、その信頼性は必ずしも高いとは言えないが、今後のさらなる技術進展を通じて、ERPなどのエンタープライズシステムに基づく円滑な業務遂行を支援する価値あるソリューションとして多くの企業への導入が進むことは間違いないと思われる。
Latest Process Mining Functionality, Challenges, and Future Evolutionary Trends
1 Latest Functions of Process Mining
Process mining tends to attract attention in terms of technology and tools, but its essence is a theoretical system and methodology (discipline) of data analysis. In fact, as the term “process” mining suggests, it can be considered as a type of data mining. However, unlike data mining, which is a broad concept that targets all kinds of events for analysis, process mining literally targets “processes” for analysis. The basic use of process mining is “process visualization,” and the visualization of processes facilitates the discovery of problems associated with the target processes. As a result, it can play a significant role in process improvement efforts.
1.1 Current Major Functions
As mentioned above, the research of process mining has started from the establishment of the methodology of “process visualization” and the development of tools. It is a function to automatically create a flowchart showing business procedures based on data extracted from IT systems used for business execution, and is called “Process Discovery. Since then, various functions have been implemented as research has progressed and tools have become more sophisticated. The following are the main analysis functions implemented in most of the current process mining tools.
Process Discovery
automatically create a flowchart of business procedures and calculate the frequency of work and time required.
Conformance Checking
compares and analyzes the current process (as-is) discovered based on data with the standard process (to-be), and extracts deviations from the current process.
Dashboards
A function to display the results of aggregation and analysis of target processes from various perspectives in various graphs and tables.
1.2 Latest Functions
In addition, in recent years, the most advanced process mining tools have begun to include the following latest functions.
Business Rule Mining
When there is a flow branching (decision node) in a target process, it automatically discovers the criteria (business rules) that determine the routing based on the data.
Simulation (What-If Analysis)
Simulate how much improvement can be expected by eliminating or automating some of the tasks in the current process visualized by the process discovery function.
Operational Support
For projects that are currently in progress, the system absorbs data related to business execution in real time, detects deviations in business operations, predicts future problems, and alerts the person in charge, suggests the best course of action, or automatically implements improvement measures.
Of the three latest functions mentioned above, business rule mining and simulation analyze past data, i.e., data that has already been completed, while operational support focuses on supporting smooth business execution by sequentially processing data related to unfinished projects. In this sense, it can be said that operational support is a form of IT solution that goes beyond the framework of analysis methodology. For this reason, Ceronis, the largest company in the process mining industry, calls this function “EMS (Execution Management System).
2 Issues to be overcome to make process mining better to be used
As seen in the acquisition of Signavio, a major tool vendor, by SAP and myInvenio by IBM, process mining is increasingly recognized as an important tool that is part of IT solutions. However, there are issues that need to be overcome in order for it to be used properly in business practices and to bring results. In this section, I would like to present the main issues from two perspectives.
2.1 Difficulties in data preprocessing
In data mining, it is said that about 80% of the total time required is spent on data preprocessing such as data collection, extraction, and cleaning. The same is true for process mining. It takes a lot of effort to properly integrate dozens to hundreds of data files extracted from various IT systems, to correct dirty data such as omissions and garbled characters, and to create a “data set” that can be fed into tools for analysis. Factors that make data pre-processing in process mining difficult include the fact that the source of data extraction is various business systems, and thus an understanding of the business systems is necessary. In addition, in order to create a data set to derive analysis results that contribute to business process improvement, it is necessary to understand the business itself and to have some familiarity with business improvement methods.
2.2 Analysis quality of tools
There are two issues that need to be addressed regarding the quality of analysis. One is the limitation of DFGs (Directly Follows Graphs), and the other is the Convergence/Divergence problem.
2.2.1 Limitations of DFGs
The basic function of process mining, “process discovery,” was initially based on Petri nets, but various algorithms have been developed to reproduce flowcharts closer to reality. However, according to industry experts, most of the process mining tools currently in practical use are said to be based on an algorithm called fuzzy miner (each company is believed to have made its own improvements).
This algorithm is commonly called DFGs (Directly-follows Graphs). Unlike Petri nets and BPMN (Business Process Modeling and Notation), which is the world standard for describing business procedures as flowcharts, DFGs are flowcharts in which nodes are directly connected to each other (directly). In other words, since branching nodes are not drawn, the algorithm cannot grasp where and how the branching is occurring, specifically, whether it is exclusive (OR) or concurrent (AND). For this reason, even if the current process is automatically reproduced, the reality is that the branching is not clear and incomplete. Of course, functional improvements have been made in this regard, such as automatic conversion to BPMN format flowcharts and the adoption of business rule mining as mentioned above.
2.2.2 Convergence/Divergence Problem
In process mining, three items, “case ID,” “activity (event),” and timestamp, are essential to draw a flowchart by bundling each activity performed for a case processed in the target process. For example, in the case of an invoice processing process, the individual invoice number attached to each invoice and the activities such as “receipt,” “confirmation,” “approval,” and “payment” for that invoice are extracted from the IT system along with the time stamp.
What we often face in the actual process is that there is no single case ID. Let’s take a concrete example. The figure below shows a general image of the process of an engineering company from order receipt to material procurement.
Since the ordered machine must be manufactured based on the specifications of the ordering company, after receiving the order, the company first designs the machine, then identifies the necessary materials and parts based on the blueprint, and then places an order with the supplier. Since multiple blueprints are created for a single machine, the Blueprint Number is used in the design stage. In addition, the Parts Number is used to identify materials and parts, and at the time of procurement, multiple parts are combined into several parts and a procurement request is issued. In this case, a Procurement Request Number is assigned. In addition, the multiple procurement requests are aggregated to each supplier and an order is placed. In this case, the Order Number becomes the ID for management.
In this way, the processes of convergence and divergence are commonly seen in practice as a single case is processed. In the conventional approach, the construction number at the beginning of the process is used as the case ID, and the entire process is analyzed up to the procurement of materials, but if there is convergence or divergence in the process, a process that is far from the actual situation is reproduced. (For example, the diffused part is recognized as a mere repetitive task.)
This Convergence/Divergence problem is the biggest issue that affects the analysis quality of process mining. In recent years, researchers led by Professor Wil van der Aalst, the Godfather of Process Mining, have been working on solving this problem using a unique methodology called “Object-Centric Process Mining” .
3 Future Direction of Evolution
We have already mentioned that process mining is playing a role as a business support solution beyond the framework of data analysis. In this section, we will discuss how process mining will evolve in the future from a bird’s eye view.
3.1 Process Mining 1.0
Process mining is. The basic function of process mining was “process discovery,” which automatically reproduces the current process from data. This is a “Descriptive Analysis” in that it depicts the current state as it is.
However, what we originally wanted to do was to extract problem areas such as inefficiencies and bottlenecks hidden in the process. In other words, we need to find out what is wrong with the process. Therefore, there is an additional function that can easily tell us where the problem is, such as the processing time of this part is too long or there are too many repetitions. This is a function that belongs to Diagnostic Analysis. In process mining tools, it is generally named “Root Cause Analysis.
The above is an analysis function for historical data, and should be called Process Mining 1.0.
3.2 Process Mining 2.0
When process mining starts to take in uncompleted, i.e., ongoing, case data in real time as a target of analysis, it becomes possible not only to detect deviations but also to predict how long it will take to complete the currently running case, and to predict deviations that may occur in the future. In addition, it is possible to predict how long it will take to complete a case that is currently running, and to predict future deviations. The number of tools that implement such predictive analysis is increasing.
Furthermore, based on the prediction results, tools that can suggest what actions should be taken now to shorten the time required or to prevent future deviations from occurring are also emerging. This is the function of “Prescriptive Analysis”.
Such process mining analysis that deals with incomplete data is a major upgrade of the existing process mining 1.0, and can be called process mining 2.0.
Although predictive and prescriptive analyses are still in their infancy and their reliability is not necessarily high, it is certain that they will be introduced to many companies as valuable solutions to support smooth business execution based on enterprise systems such as ERP through further technological progress in the future.